
Integration of PBPK/PD Models to Inform Compound Design and Prioritization in Early Stages of Drug Discovery
-
Register
- Non-member - $49
- Member - Free!
- Student - Free!
- Premier - Free!
Physiology-based pharmacokinetic (PBPK) models are broadly applied in late phase drug discovery/ development. Traditionally, compounds are prioritized based on hierarchical filtering with predefined cut-offs for desirable range of various parameters. PBPK models, when combined with related pharmacodynamic assumptions, offer a valuable platform to integrate multiple parameters driving the in vivo PK profile required for target engagement. They also provide mechanistic interpretation of key drivers for the predicted profile to further help with compound design strategies. As such, these results enable compound prioritization in a holistic manner, focusing on multi-property optimization (MPO). This presentation will provide a brief overview of the structure and application of an internal PBPK model. Examples of successful application of this tool on a small molecule drug discovery program will be shared to illustrate its role in driving decisions to guide compound progression.
The optimal PK necessary for a drug candidate to achieve efficacy is highly dependent on the targeted PD, a relationship often not well characterized during the early stages of drug discovery. Relying on generic assumptions about PK and potency can misguide screening and compound design, leading to suboptimal ADME or molecular properties. This, in turn, may increase attrition rates and extend hit-to-lead and lead optimization timelines. The "PD before PK" approach, detailed in this presentation, can be applied in two ways: forward, to virtually screen compounds for potential effectiveness, and reverse, to identify pharmacology-specific PK drivers and the related potency-ADME space early in discovery. This strategy aims to enhance the probability of success and reduce clinical attrition. Potential applications of this approach will also be discussed.
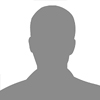
Emile Chen
Dr. Emile Chen has thirty years of industrial experience divided between early discovery involved in lead optimization and candidate selection, and late-stage development, including authoring and reviewing of regulatory documentation and NDA submission. Until March 2024, he was in the System Modeling and Translational Biology group, using PBPK, Mechanistic PKPD modeling, QSP, and machine learning techniques to solve project questions and thereby enhance scientific productivity.
Emile received his undergraduate degree from University of California at Los Angeles and his PhD from Northwestern University in the field of Biomedical Engineering, specializing in developing mathematical models for the information processing in the brain. He began his pharmaceutical carrier at Hoffmann La-Roche in 1993, after completing a postdoctoral fellowship in University of California at San Francisco. He joined GlaxoSmithKline in1996. Over the years, he has led ADME and PK groups at various times in supporting either early discovery or late development DMPK efforts. More recently, recognizing the current challenge to improve R&D productivity for the pharmaceutical industry, Emile is focused in leading efforts to use innovative mathematical modeling and simulation methods to help reduce attritions while enhancing ability to predict efficacy and safety in human and support portfolio investment decisions. For the past 10+ years, he also designed and teaching a series of interactive workshops that promote the use of kinetic thinking and mathematical modeling to integrate preclinical and clinical information to aid decision making during drug discovery and development. The workshops are offered several times each year both internally and externally.
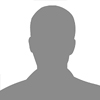
Wenyi Wang
Dr. Wenyi Wang is a Principal Scientist in the In Silico predictive ADME (pADME) group within the DMPK function at Genentech. She received her PhD from Rutgers University in application of machine learning for addressing challenges in ADME-Tox. In her current role at Genentech she actively engages with portfolio teams to build and apply ML and mechanistic PK models to address ADME related issues and inform prioritization for compound synthesis and testing.
Key:




